Description
The task is to perform blocking for Entity Resolution, i.e., quickly filter out non-matches (tuple pairs that are unlikely to represent the same real-world entity) in a limited time to generate a small candidate set that contains a limited number of tuple pairs for matching.
Participants are asked to solve the task on two product datasets. Each dataset is made of a list of instances (rows) and a list of properties describing them (columns). We will refer to each of these datasets as Di.
For each dataset Di, participants will be provided with the following resources:
- Xi : a subset of the instances in Di
- Yi : matching pairs in Xi x Xi. (The pairs not in Yi are non-matching pairs.)
- Blocking Requirements: the size of the generated candidate set (i.e., the number of tuple pairs in the candidate set)
Note that matching pairs in Yi are transitively closed (i.e., if A matches with B and B matches with C, then A matches with C). For a matching pair id1 and id2 with id1 < id2, Yi only includes (id1, id2) and doesn't include (id2, id1).
Your goal is to write a program that generates, for each Xi dataset, a candidate set of tuple pairs for matching Xi with Xi. The output must be stored in a CSV file containing the ids of tuple pairs in the candidate set. The CSV file must have two columns: "left_instance_id" and "right_instance_id" and the output file must be named "output.csv". The separator must be the comma. Note that we do not consider the trivial equi-joins (tuple pairs with left_instance_id = right_instance_id) as true matches. For a pair id1 and id2 (assume id1 < id2), please only include (id1, id2) and don't include (id2, id1) in your "output.csv".
Solutions will be evaluated over the complete dataset Di. Note that the instances in Di (except the sample Xi) will not be provided to participants. More details are available in the Evaluation Process section.
Both Xi and Yi are in CSV format.
Example of dataset Xi
instance_id | attr_name_1 | attr_name_2 | ... | attr_name_k |
---|---|---|---|---|
00001 | value_1 | null | ... | value_k |
00002 | null | value_2 | ... | value_k |
... | ... | ... | ... | ... |
Example of dataset Yi
left_instance_id | right_instance_id |
---|---|
00001 | 00002 |
00001 | 00003 |
... | ... |
More details about the datasets can be found in the dedicated Datasets section.
Example of output.csv
left_instance_id | right_instance_id |
---|---|
00001 | 00002 |
00001 | 00004 |
... | ... |
Output.csv format: The evaluation process expects "output.csv" to have 3000000 tuple pairs. The first 1000000 tuple pairs are for dataset X1 and the remaining pairs are for datasets X2. Please format "output.csv" accordingly. You can check out our provided baseline solution on how to produce a valid "ouput.csv".
# | Name | Description | Number of rows | Blocking Requirements | Download Sample |
---|---|---|---|---|---|
1 | Notebook | Notebook specifications | About 1000000 | Candidate Set Size = 1000000 | Dataset X1 Dataset Y1 |
2 | ![]() |
Product specifications Kindly provided by Altosight |
About 1000000 | Candidate Set Size = 2000000 | Dataset X2 Dataset Y2 |
Quick start:
Participants are suggested to start with our provided baseline solution.
Submission:
Each team can make at most 10 submissions each day. The number of remaining submissions will be reset at 11:59:59 PM Eastern Standard Time.
Participants are asked to use ReproZip to pack the solution they want to submit.
ReproZip is a tool for packing input files, libraries and environmental variables in a single bundle (in .rpz format), that can be reproduced on any machine.
A brief guide on how to use ReproZip to package your solution follows.
First of all, you have to install ReproZip on your machine. ReproZip can be installed via pip (pip install reprozip). More details about the installation can be found in the dedicated Documentation page.
Let’s suppose that your code is made up of a Python module called "blocking.py" and that you launch your program with the following command: python blocking.py.
First of all, ReproZip needs to track the code execution. For this to happen, it will be sufficient to run the following command: reprozip trace python blocking.py.
The code will be executed and a hidden folder (called ".reprozip-trace") will be created at the end of the process. This folder contains a "config.yml" file, that is a configuration file containing information about input/output files, libraries, environmental variables, etc. traced during the execution of your code. If you want to omit something you think it’s not useful to be packed, you can manually edit this file. Please, be sure not to remove any libraries or files needed to reproduce the code, to avoid the risk of it being not-reproducible.
Finally, to create the bundle, you have to run the following command: reprozip pack submission.rpz.
Please note that, if your code is made up of more than one file, even if they are written in different programming languages, you can trace the execution by using the option "--continue". You can find more details in the dedicated Documentation page.
At this point, a file called "submission.rpz" will be created and you can submit it using our dashboard.
Inside your code, it is important to refer to each input dataset Xi using its original name "Xi.csv".
Submitted solutions will be unpacked and reproduced using ReproUnzip on an evaluation server (Azure Standard F16s v2) with the following characteristics:
Processor | 16 CPU x 2.7 GHz |
Main Memory | 32 GB |
Storage | 32 GB |
Operating System | Ubuntu 20.04.3 LTS |
In particular, before to run ReproUnzip, the Xi dataset (i.e., the original input you worked on) will be replaced with the complete dataset Di, which contains the hidden instances.
Here is the detailed sequence of operations used for the evaluation process:
- reprounzip docker setup <bundle> <solution>, to unpack the uploaded bundle
- reprounzip docker upload <solution> Di.csv:Xi.csv to replace the input datasets (X1.csv, X2.csv, ...) with the complete ones (D1.csv, D2.csv, ...)
- reprounzip docker run <solution>
- reprounzip docker download <solution> output.csv (i.e., you must produce just one file "output.csv" cumulative for all the datasets)
- evaluation of "output.csv"
Important Notes: Your solution will be evaluated on Di, but in order to be evaluated, your submission must meet the following requirements:
- The program must be reproduced correctly (i.e., the process must end with the creation of the "output.csv" file without errors).
- The program must be finished within 35 min otherwise it incurs "timeout" error (i.e., the total time limit for blocking on two datasets is 35 min).
- The size of the candidate set (i.e., the number of rows in the output.csv) must equal to the size specified in the blocking requirements of Di.
Evaluation Metrics: For each dataset Di, we will compute resulting recall score as follows: $$Recall = {\text{Number of true matches retained in the candidate set} \over \text{Total number of true matches in ground truth}}$$ Note that the trivial equi-joins (tuple pairs with left_instance_id = right_instance_id) are not considered as true matches. Submitted solutions will be ranked on average Recall over all datasets. Ties will be broken with running time. Note running time may vary slightly when submitting the same solution multiple times. After the final submission deadline, we will run solutions with ties on recall ten times to get averaged running times.
Unfortunately, ReproUnzip sometimes prints useful information about the occurred errors on stdout, causing its exclusion from the submission log. In case you are stuck on a technical error preventing the successful reproduction of your submission, with no useful information appearing in the log, and not even the provided ReproUnzip commands can help you to find out the cause of the error, you can send us an email, so that we can check the content of stdout in order to detect the presence of any useful information about the error.
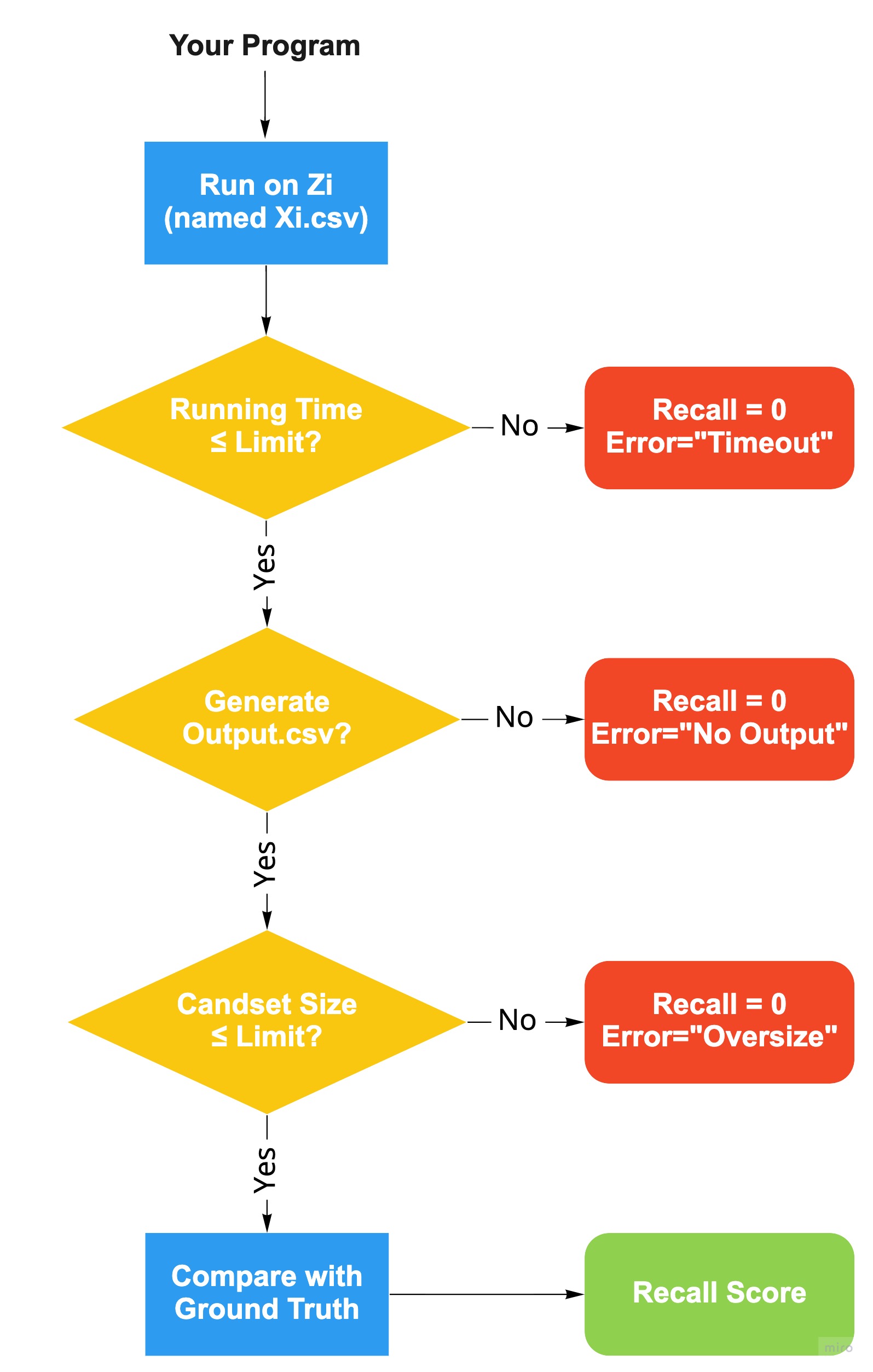